Astronomical Researchers Uncover 562 Fresh Prospective Strong Gravitational Lenses Utilizing Machine Learning
Through an international research partnership, computers were educated to sift through millions of images in search of cosmic treasures.
Perched 7,200 feet above sea level on a mountaintop in Chile, the unfolding cosmos is captured frame by frame. The Cerro Tololo Inter-American Observatory houses the 4-meter Blanco Telescope within a colossal dome, where scientists have been scrutinizing the celestial expanse since 1976. Despite technological advancements generating an exponentially increasing amount of data, a significant hurdle remains: the speed at which individuals can sift through this vast trove.
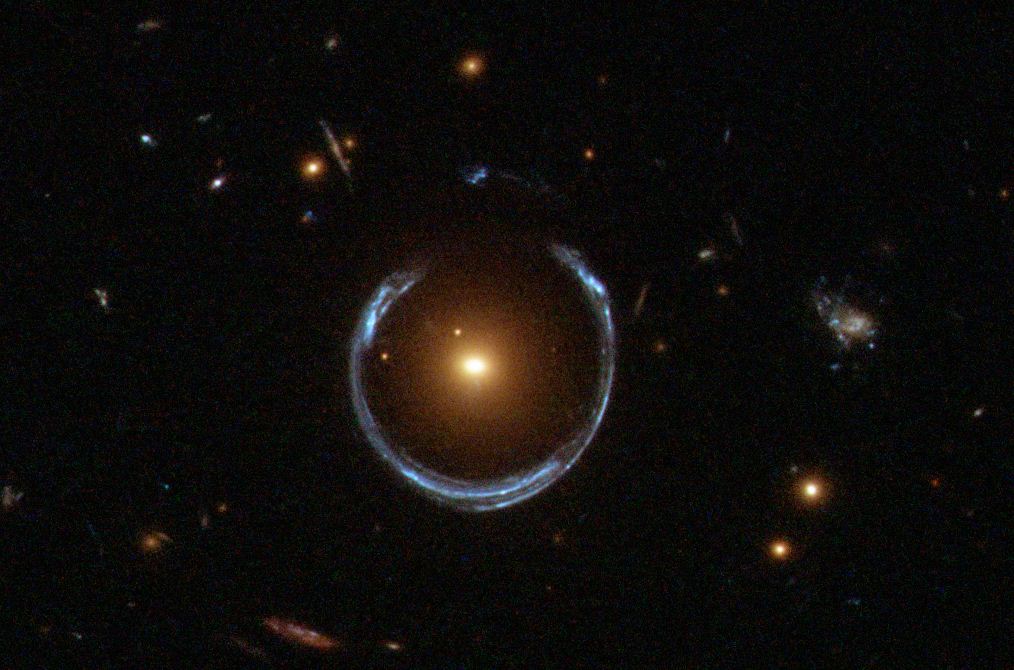
ESA / Hubble & NASA
A collaborative effort among international astronomers has yielded a machine-learning model tailored to analyze images from the telescope, specifically to identify faint galaxies. Employing this model, researchers efficiently narrowed down 11 million images—all sourced from the southern sky—to a mere 581 showcasing a phenomenon known as strong lensing.
When a colossal cosmic entity positions itself in front of a more distant galaxy, its gravitational force distorts and warps the background galaxy’s light. This gravitational lensing not only magnifies the background galaxy, exposing previously unseen features, but also often contorts it into an elongated image. Such prominently lensed galaxies provide astronomers with the means to uncover insights into the history and evolution of our universe that would otherwise remain imperceptible.
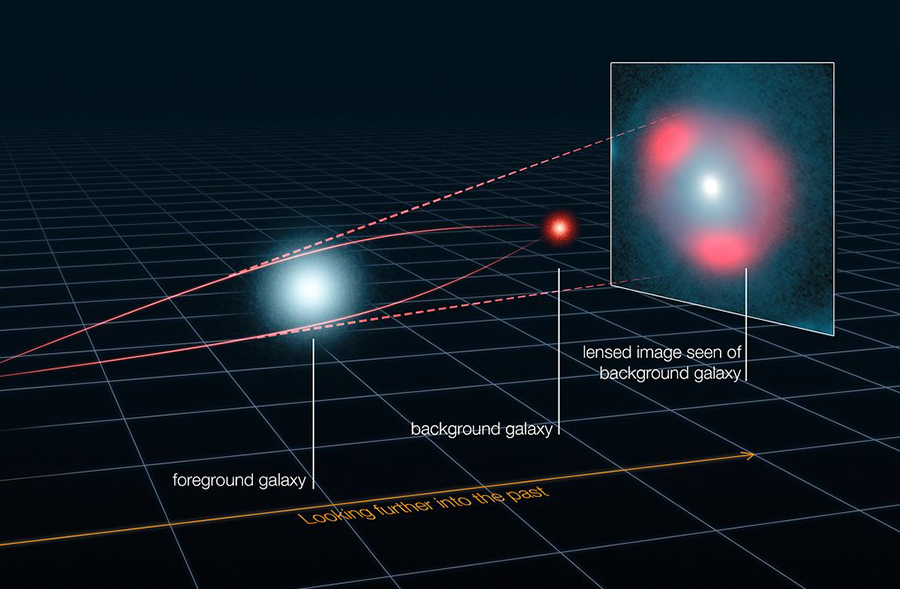
ALMA (ESO / NRAO / NAOJ) / L. Calçada (ESO) / Y. Hezaveh et al., with modifications
Altogether, from the vast number of galaxies already documented, astronomers have officially verified approximately 1,000 as being gravitationally lensed. “Strong lenses are genuinely scarce,” remarks graduate student Erik Zaborowski from Ohio State University, the leader of the study published in the September 1st Astrophysical Journal. As observatories like the DECam-equipped Blanco Telescope unveil the southern sky for exploration, astronomers are anticipated to uncover concealed lenses in this relatively unexplored celestial region. “It’s really nice to have this really unsearched area of the sky become available now,” he adds.
Strongly lensed galaxies play a pivotal role in addressing some of astronomy’s paramount inquiries, spanning from the nature of dark matter to the universe’s expansion rate. Yet, with a predominant focus on astronomical searches in the Northern Hemisphere, only recently have astronomers methodically surveyed the entirety of the southern sky.
Facilitating this endeavor is the Blanco Telescope’s Dark Energy Camera (DECam), operational since 2012. Engineered to capture expansive celestial expanses in a single image—equivalent to an area the size of 20 Moons as seen from Earth—the DECam enhances the capability to survey more sky.
As the volume of sky for examination increases, the data becomes overwhelming for manual analysis. “The amount of data that we’re going to have to sift through is going to be impossible for a human to go by eye,” notes Greg Mosby from NASA Goddard, who utilizes machine-learning algorithms in his own research.
This is where machine learning, a burgeoning facet of artificial intelligence, comes into play. Computer algorithms in machine learning are trained through data rather than direct human intervention, and they are increasingly utilized in diverse fields such as language translation, targeted advertising, and image identification—now gaining traction in astronomy.
Zaborowski and his team pioneered the application of machine learning to public data from the DECam Local Volume Exploration Survey (DELVE), which commenced in 2019 and has documented an impressive 520 million cosmic sources. From this vast dataset, the team selected 11 million extended sources and employed a five-layered convolutional neural network, a prevalent machine-learning technique for image classification, to search for strongly lensed galaxies.
Prior to the network identifying actual lensed galaxies, it underwent training. Over 80,000 real galaxy images from DELVE were used, with an artificial lensing effect added to half of them. Additionally, more than 3,200 false positives—images that resembled potential strongly lensed galaxies but were not—were included to refine the model’s accuracy.
Human involvement remained integral, with the model generating 50,000 sources deemed most likely to be lenses. Subsequently, scientists manually examined each one. The outcome was 581 extended sources identified as probable strongly lensed galaxies, including 562 previously unreported instances. Furthermore, eight potentially lensed quasars, highly luminous galaxy cores, were discovered. Pending further scrutiny, these new lensed galaxies could augment the total count by over 50%.
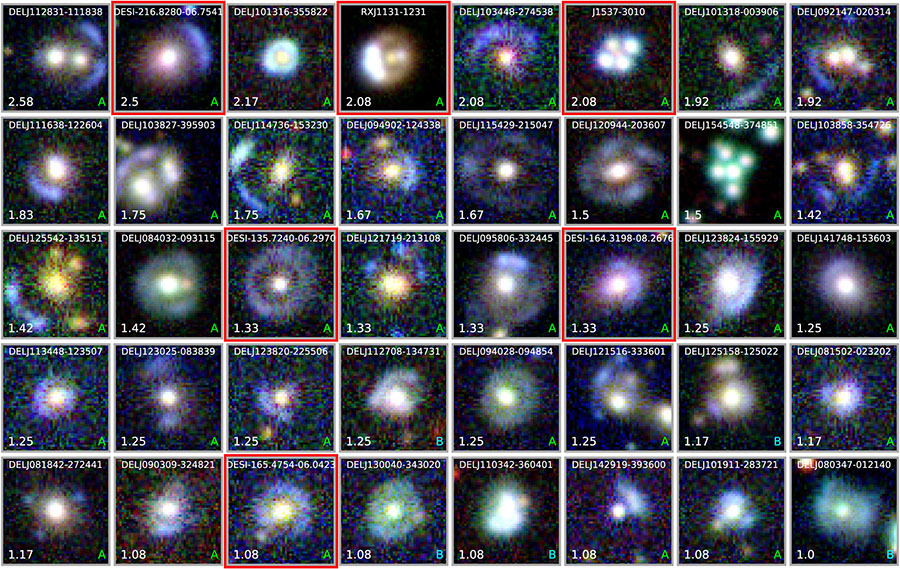
Zaborowski et al. / Astrophysical Journal 2023
While the abundance of new potential strong lens candidates presents a significant advancement, it prompts astronomers to reflect on the implications of this new era characterized by vast databases and machine-learning models. One notable consideration is that the efficacy of these models is contingent upon the quality of the training data. Given the scarcity of authentic images of strong lenses, scientists are compelled to train models using synthetic ones.
Expressing reservations, Ben Metcalf from the University of Bologna in Italy, who was not part of the study, highlights the inherent challenge: if real data contains elements not represented in simulated data, the model’s response to such elements remains unknown. Essentially, while the model may excel at identifying typical strong lenses, there is a risk of overlooking distinctive instances. Greg Mosby echoes this concern, suggesting ongoing human involvement in the search until machine-learning models can be trained on rarer lenses.
A related constraint lies in the subjective nature of astronomers’ perceptions of what visually qualifies as a strong lens. Establishing a consensus among scientists is imperative to guide models on the criteria for selection.
Despite machine learning’s inherent limitations, its forte lies in speed. Tasks that would consume months for researchers to complete in tedious image classifications can be accomplished by models within mere hours. With an ever-expanding pool of data at our disposal, this study underscores the potential of machine learning to propel us to the next phase: unraveling the insights that strong lenses offer about the universe.
Erik Zaborowski emphasizes the significance of this data, stating, “There are a lot of kinds of analyses that you can really only do with a lot of these [lenses]. With that data, you can start answering the science questions.”
This article is republished from SkyandTelescope under a Creative Commons license. Read the original article.
Do not forget to share your opinion with us to provide you with the best posts !
0 Comments